Deep Learning – Mimicking the Human Brain for Smarter AI
- Fitzroy Petgrave
- Oct 24, 2024
- 5 min read
Updated: Oct 25, 2024
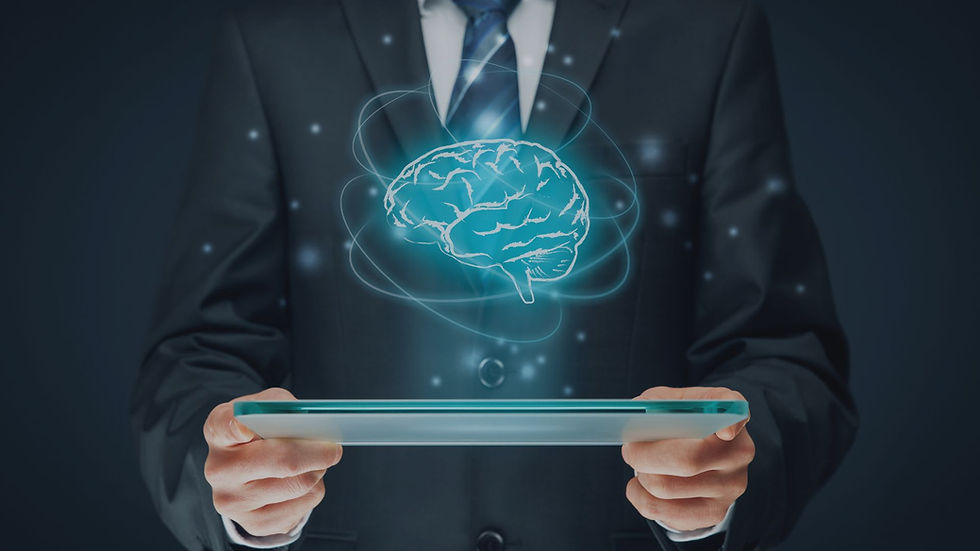
By now, you’ve likely heard of machine learning (ML) and its role in helping businesses make smarter decisions with data. But if you’re following advancements in AI, you may have encountered the term deep learning. While deep learning is a subset of machine learning, it operates on a different level, often compared to how the human brain works. But what does that really mean?
In this post, we’ll explore deep learning in simple, relatable terms. We’ll also look at how it differs from traditional machine learning and why it’s such a game-changer for businesses tackling complex challenges like understanding unstructured data, recognizing images, and even revolutionizing waste management processes.
What is Deep Learning?
To put it simply, deep learning is an advanced form of machine learning. If machine learning is about teaching a computer to learn from data, deep learning is like teaching the computer to think more like us. The key difference lies in the depth of the learning process.
Let’s use a human brain analogy, which is often the easiest way to understand deep learning. Just like your brain is made up of billions of neurons that work together to process information, deep learning uses artificial neural networks. These networks are mathematical models inspired by how neurons in our brains connect and communicate. The deeper the network, the more complex its understanding becomes.
How Does Deep Learning Work?
Imagine deep learning as a layered system, where each layer is responsible for processing data in a more sophisticated way. These layers act like “neurons” in a brain, where the input layer receives raw data (such as text, images, or sound), and the output layer produces results (like identifying an object in an image or recognizing spoken words).
In between the input and output layers are several hidden layers. These hidden layers transform the raw input into something meaningful by detecting patterns. For instance, if a deep learning model is analyzing an image of a plastic bottle, the first layer might detect the basic shape. Deeper layers may then identify details like texture or color, and eventually recognize it as a plastic bottle.
This multi-layered approach is why it’s called deep learning—the model gets better at understanding and learning as it moves through multiple layers of abstraction.
Deep Learning vs. Machine Learning: What's the Difference?
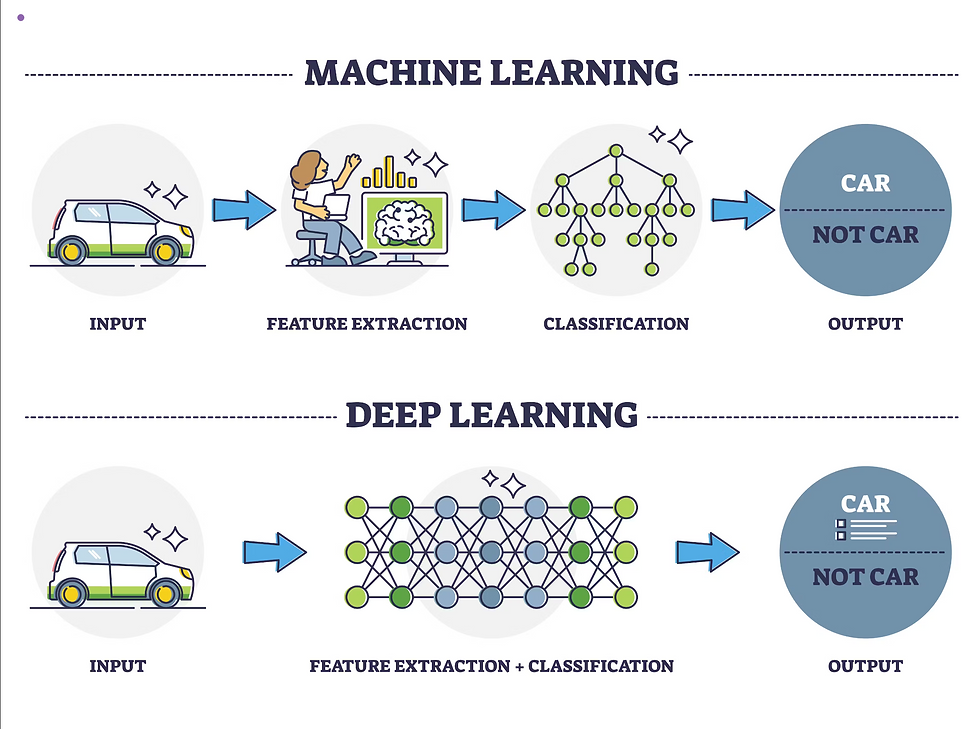
Although deep learning is a part of machine learning, they differ in complexity and autonomy.
Machine Learning: In traditional machine learning, a human often guides the model by telling it what to look for in the data. For example, if you’re training a machine learning model to identify recyclable waste, you might start by giving it features to detect, like shape, material type, or size. The model will use these instructions to make predictions based on the data you give it.
Deep Learning: Deep learning models, on the other hand, don’t require this level of human intervention. They can analyze raw data and figure out the patterns on their own. For instance, in a deep learning model, you don’t need to tell the AI to look for the shape or material type. You simply give it thousands of images of recyclable and non-recyclable items, and the model learns on its own which characteristics are important. This makes deep learning much more powerful for handling complex tasks like image recognition or natural language processing.
Why is Deep Learning So Powerful?
Deep learning’s power comes from its ability to process massive amounts of complex data without needing a human to define what to look for. Here’s where deep learning excels in real-world applications:
Image Recognition: Deep learning models are great at identifying objects in images. This can be used in recycling facilities to automatically classify different types of waste, such as glass, plastic, or metal, by analyzing visual patterns.
Natural Language Processing (NLP): Virtual assistants like Siri or Alexa can understand spoken requests because deep learning is used to interpret and process speech. For businesses, this means being able to automate responses to customer queries or handle voice-activated commands.
Automation: From autonomous vehicles to smart recycling systems, deep learning enables machines to learn from data and perform tasks without human input, improving efficiency and reducing costs.
Real-World Analogy: Sorting Waste in a Recycling Facility
Let’s bring this closer to the world of waste management. Imagine a recycling facility where workers manually sort through piles of trash to separate materials - plastic bottles, cardboard, metal cans, etc. At first glance, they may recognise the general types of items by their shape or colour. Over time, however, they get faster and more accurate, identifying even subtle differences, like distinguishing between a type of plastic that can be recycled and one that can’t.
Now imagine that same facility using a deep learning model. Instead of teaching the model to recognize individual materials by their basic features (as with traditional machine learning), you feed the model a large amount of data - images of different items being sorted. Over time, the AI becomes better and better at automatically recognizing and sorting the items, even when they appear in different sizes, conditions, or lighting.
This is where deep learning shines: it learns from experience, getting smarter and more precise the more data it processes. Just as a human worker becomes more efficient through experience, a deep learning model becomes more adept at recognizing and sorting different types of waste by analyzing more and more data.
Deep Learning Challenges: Why Isn’t Everyone Using It?
While deep learning sounds revolutionary, it comes with its own set of challenges.
Data Requirements: Deep learning models need vast amounts of data to perform well. In the context of waste management, you would need a large dataset of correctly labeled images of recyclable and non-recyclable materials for the model to learn effectively.
Computational Power: Training deep learning models requires heavy computational resources, including specialized hardware like GPUs (Graphics Processing Units). For many businesses, especially smaller ones, this can be expensive and may require cloud-based solutions.
Complexity: Deep learning models can be very complex, making them difficult to interpret. This “black box” nature means you may not always understand why the model made a certain decision, which could be problematic in highly regulated industries like healthcare or finance.
How Can Deep Learning Benefit Your Business?
Despite these challenges, the potential benefits of deep learning for businesses—especially those involved in waste management—are immense:
Increased Efficiency: In a waste management context, deep learning could automate the sorting of recyclables, reducing the need for manual labor and speeding up operations.
Improved Accuracy: With deep learning, the system would get better at recognizing materials over time, improving the quality of the sorting process and reducing contamination.
Cost Reduction: By automating more tasks, deep learning can reduce operational costs. Fewer mistakes in sorting mean fewer fines for contamination or fewer rejected loads at recycling plants.
Why Deep Learning Matters
Deep learning is more than just a buzzword in AI - it’s a powerful tool that can transform industries. From recognizing recyclable materials in waste management to enabling more personalized customer experiences in e-commerce, deep learning allows businesses to leverage vast amounts of data in new, innovative ways.
In our next post, we’ll explore natural language processing (NLP) - another area where deep learning shines - and how it enables machines to understand and respond to human language, offering exciting possibilities for customer engagement and automation. Stay tuned for more insights!
Comments